Using personal data in AI projects: Overcoming the challenges
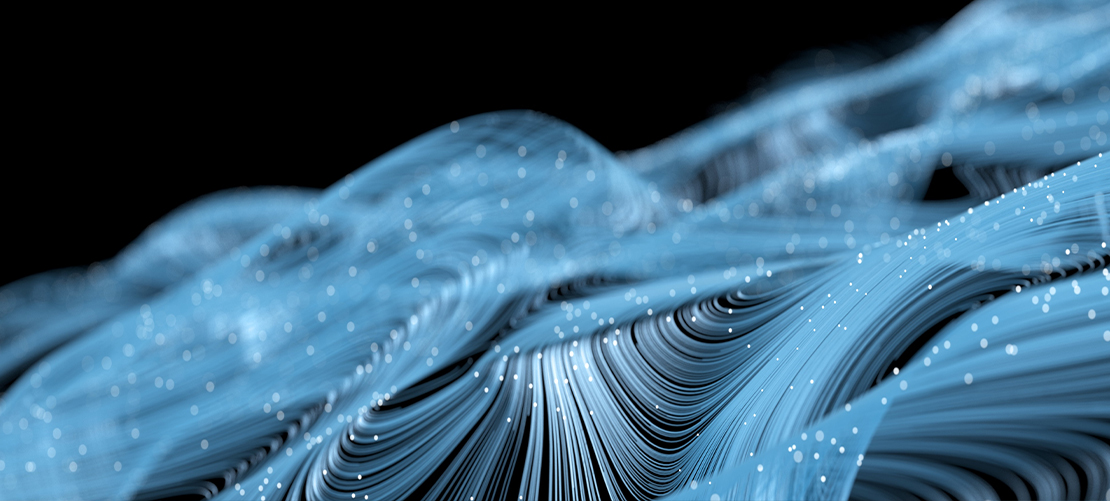
Be transparent, and to be on a safe side, conduct a DPIA whenever AI is involved. By Katie Hewson and Kate Ackland of Stephenson Harwood.
It has been reported this month that the number of generative AI users in China has surpassed a huge 600 million1 and more than 200 generative AI service models have been registered in the country. Quite an impressive feat. This year, AI has made leaps and bounds in revolutionising technological advances across industries including coral reef restoration, personalised curriculums and bespoke treatment plans for patients. Even the European Commission has launched its own generative AI tool to help staff with generating policy documents. Whilst the opportunities presented by AI tools seem to be infinite, it certainly does not come without its challenges. According to a report2 from Boston Consulting Group, 33% of consumers using AI were most concerned about data security and ethics. Given our increasing awareness of how our data is being used and exploited in this rapidly evolving technological world, this key concern is hardly surprising.
Data (including personal data) is the fundamental ingredient for the development of a successful AI tool, particularly in the training phase. Where personal data is involved, there is a delicate balance to be struck between the thirst for innovation and the strictly regulated use of personal data. This is becoming increasingly clear as more and more organisations have expressed concern around debilitating regulations to their desires for invention. It was expressed rather bluntly in an open letter3 to EU policymakers signed by 49 companies and researchers including the CEOs of Meta, Spotify, SAP and Ericsson. The letter describes regulatory decision-making in the AI and personal data sphere as “fragmented and unpredictable” with “huge uncertainty about what types of data can be used to train AI models”.
The regulators' perspective
So what challenges are organisations facing? It is helpful to consider some of the recent reprimands issued by data protection regulators that demonstrate the key areas of focus in this realm of AI and personal data. It comes as no surprise that these reprimands have, so far, arisen mainly with the biggest technology platforms in the world such as LinkedIn, X, Google and Meta.
LinkedIn recently suspended its use of UK member content and data to train its AI models after the ICO expressed concerns around the lack of transparency for users saying in a statement “it is crucial that the public can trust that their privacy rights will be respected from the outset”4. Earlier this year, X agreed to limit its use of personal data contained in public posts of European users to train its AI models following commencement of court proceedings against X by the Irish Data Protection Commission (DPC). The key issues raised in the proceedings were that the purposes for using the personal data were not sufficiently clear and that more data than was strictly necessary was being collected by X. There were also claims that sensitive data was being used without sufficient legal bases for doing so. Similar issues arose in June of this year when Meta agreed to pause certain processing of personal data following complaints against its reliance on “legitimate interest” to use data to train AI models. Google has also come under fire by the Irish DPC for failure to carry out a Data Protection Impact Assessment (DPIA) prior to engaging in the processing of personal data associated with the development of its AI model, Pathways Language Model 2.
Key challenges when using personal data for AI projects
As the regulatory landscape continues to evolve alongside the unremitting growth of AI, it is clear that there are numerous challenges to be overcome when processing personal data in the use or development of AI. We have set out below some of the most significant, as well as some checklists of practical tips on how to manage them.
Be transparent
Consider whether your privacy notices need to be updated to address your AI systems. Have you explained in a clear and concise manner how you are using personal data in the context of AI? Are you using AI to collect personal data? Are you using personal data to train your AI models? Put yourself in an individual’s shoes to assess how the information you publish may be interpreted or perceived. Is it clear enough? Is it easily accessible? Transparency has been one of the top items on the ICO’s list of issues when it comes to the use of AI.
Give individuals the ability to opt out
Whilst not always legally required, it is sensible to consider giving an individual the right to opt out of the processing of their personal data where AI is involved. This was a key area of concern for the Italian Data Protection Authority, the Garante, when it demanded the temporary suspension of ChatGPT in April 2023. Providing users with the right to opt out from having their data processed for algorithmic training or other AIrelated processing demonstrated the prioritising of the fundamental protection of data subject rights. LinkedIn recently rolled out this right in the UK, having previously switched its AI training feature on by default.
Consider the appropriate legal basis
When using personal data in AI projects, it is important to break down and separate each processing function (e.g. research and development vs. deployment of AI systems) and identify distinct purposes of the processing as well as the appropriate lawful basis for each function. It may be that there is more than one appropriate lawful basis for each function. Document your decision and make the determination before you start processing. Note that there have been multiple challenges over the reliance on “legitimate interest” as the legal basis for training AI models, so it is really important to do a legitimate interest assessment when seeking to rely on legitimate interest as a legal basis. You should also ensure that you monitor any changes to the use of AI and/or the corresponding personal data which may require a change to the lawful basis originally determined as the most appropriate.
Conduct DPIAs
As Google discovered with the development of its AI model, DPIAs are a must when it comes to the development and deployment of AI systems. The ICO’s view is that in most cases where AI is being used, it will likely result in a high risk to individuals’ rights and freedoms and therefore trigger the legal requirement to undertake a DPIA under the UK GDPR. In addition, the use of AI is likely to fall within the remit of “use of new technologies”, activities involving “evaluation or scoring”, “systematic monitoring” or “large-scale processing”, all of which trigger the requirement to conduct a DPIA. Whilst an assessment should be conducted on a case-by-case basis, it may be safer to conduct a DPIA whenever AI is involved. Equally, if a decision is made that a DPIA is not required, the decision and reasons for such a decision should be documented.
Do not use more personal data than is necessary
Under the UK GDPR, you are required to process the minimum amount of personal data necessary for the intended purpose. AI systems clearly rely on significant amounts of data so you need to determine what is “adequate, relevant and limited to what is necessary” which will always need to be determined on a case-by-case basis. Ensure that the amount of personal data being processed is reviewed at regular intervals to assess whether it is still absolutely necessary for the purposes pursued.
Keep data accurate
The UK GDPR requires you to ensure that personal data is accurate and, where necessary, kept up to date. This applies to all personal data including where it may be used as an input to an AI system or an output. The ICO makes a clear distinction between this accuracy principle and “statistical accuracy” which refers to the accuracy of an AI system itself. Note that the accuracy principle does not require the statistical accuracy of the AI system to be 100% accurate. In the context of AI systems, compliance with the accuracy principle is important – the input and the output of any AI system should contain accurate personal data. There are certain exceptions including where an output is a prediction as opposed to a statement of fact. Practical ways of achieving the accuracy principle include defining and documenting specific criteria for what the input data is and should be, documenting and analysing the impact of the input data on outputs, reviewing and investigating any inaccuracies of output data and making it clear to data subjects that outputs have been generated by AI or may not be factually accurate. This is likely to become even more pertinent given the expectations under the EU AI Act that data sets used to train certain AI models must meet specific quality criteria, including being free from errors.
Secure your AI systems
The National Cyber Security Centre has identified a number of heightened security risks in AI systems including prompt injection attacks where an attacker creates an input designed to mak the model behave in an unintended way, and data poisoning attacks where an attacker tampers with the data that an AI model is trained on to produce undesirable outcomes5. Security of AI systems should be considered at the outset of development implementing a security “by design” approach. The ICO suggests conducting security risk assessments, debugging datasets and proactively monitoring systems to identify and mitigate any anomalies. If using AI provided by a third-party vendor, ensure that appropriate information security diligence is conducted on that vendor. The appropriate measures to put in place will ultimately depend on the level and types of risk which may arise from specific processing activities.
Determine your role
Are you a controller, joint controller or a processor? Are you procuring an AI system from a third party? Are you deciding how to deploy the AI system? Are you developing your own AI systems using personal data? These are all questions to ask to help determine your role within the scope of data protection laws and therefore your obligations. Where you are controller of the personal data, you have overall accountability for data protection compliance. Note that you might be acting as a controller for specific processing functions and a processor for others.
Prioritise data subject rights
Due consideration must be given at the outset to how you comply with an individual’s request for access, rectification, erasure or other information rights. This can be challenging when AI systems are involved depending on the nature of the processing. For example, where training an AI system with a large dataset of customer transactions, how do you identify the individuals that the training data is about? You should also consider whether your outputs are labelled as predictions or statements of fact. Note that the right to rectification does not apply where the personal data is a prediction. The ICO stresses that all efforts should be made to respond even if the requests are more complicated due to the use of AI. If you are engaging a third party to develop or provide your AI system, make sure you understand how the model is trained and whether any personal data is included in the training data – you will be responsible for dealing with requests from data subjects if you are the controller.
Automated decision making
The UK GDPR provides data subjects with the right not to have solely automated decisions applied to them which have legal or other significant effects. AI systems will often involve automated decisions being taken based on personal data, and there is often no human intervention in the decision being made by the AI system. The key question will therefore be whether the AI system is to be involved in a decision that impacts individuals in a legal or similarly significant way. What kind of decision is the tool making about an individual? Is there any meaningful human involvement in reaching the decision? If there is not, do any of the exemptions from the right not to be subject to a solely automated decision apply – for example, is the decision necessary entering into a contract with the data subject? Have you informed the individual? Have you established ways in which the individual can exercise their rights to contest any such decision? It is important to ensure that this risk is considered at the outset.
It goes without saying that the above sets out just a snapshot of some of the key challenges organisations are facing when using personal data in AI projects. As expected, regulatory bodies, including the ICO, have made it clear over the past few months that they will be scrutinising use of personal data in the development of AI models. It is therefore hugely important to consider the risks and challenges as well as any mitigations carefully in the deployment of AI systems.
More experience in, and guidance on the interplay between the use of personal data in the development and deployment of AI would be a welcome addition to the current risk management landscape. However, these kinds of challenges are typical of the issues we see when slower, more restrained regulatory frameworks struggle to keep up with fast-moving technological innovation.
This was originally published in Privacy Laws & Business UK Report, November 2024, www.privacylaws.com
1 Registered users of large generative AI models in China exceed 600 million, data shows - Global Times,
www.globaltimes.cn/page/202410/1321127.shtml
2 Consumers Know More About AI Than Businesses Think, BCG https://www.bcg.com/publications/2024/consumers-know-more-about-ai-than-businesses-think
3 Ensuring AI innovation in Europe: Open letter to EU policymakers (euneedsai.com) www.euneedsai.com/#signatories
4 Our statement on changes to LinkedIn AI data policy, ICO ico.org.uk/about-the-ico/mediacentre/news-and-blogs/2024/09/ourstatement-on-changes-to-linkedin-aidata-policy/
5 AI and cyber security: what you need to know, UK National Cyber Security Centre, http://www.ncsc.gov.uk/guidance/aiand-cyber-security-what-you-need-toknow